- 수업 내용 리마인드 및 아카이빙 목적의 업로드
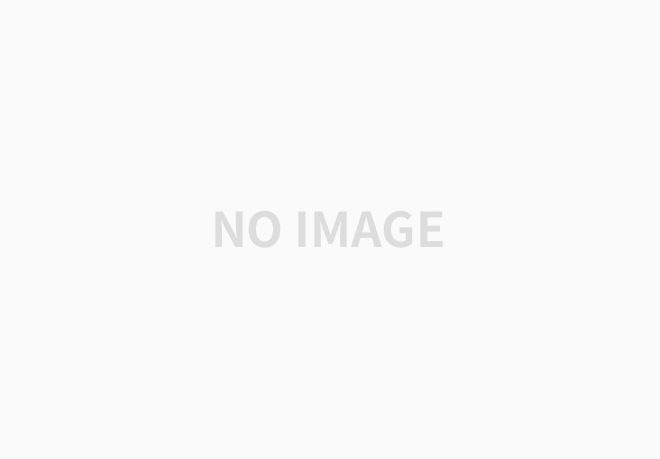
1. Twenty Newsgroups 데이터를 통한 LDA 분석 (Gensim)
Twenty Newsgroups 데이터셋은 사이킷런에서 제공하는 20개의 서로 다른 주제를 가진 뉴스 그룹 데이터를 포함하고 있습니다. 이 데이터셋을 활용하여 LDA 분석을 수행해보겠습니다.
1) 필요 모듈 로드
먼저, 데이터 전처리와 모델링에 필요한 라이브러리를 로드합니다.
import pandas as pd
from sklearn.datasets import fetch_20newsgroups
import nltk
from nltk.corpus import stopwords
from sklearn.feature_extraction.text import TfidfVectorizer
from gensim import corpora
import gensim
2) 데이터 로드
Twenty Newsgroups 데이터셋을 로드하고 기본 정보를 확인합니다.
# 데이터셋 로드
dataset = fetch_20newsgroups(shuffle=True, random_state=1, remove=('headers', 'footers', 'quotes'))
documents = dataset.data
# 샘플 수 확인
print('샘플의 수 :', len(documents))
# 첫 번째 문서 출력
print(documents[1])
# 타겟 이름(주제) 출력
print(dataset.target_names)
3) 데이터 전처리
텍스트 데이터를 모델에 입력하기 전에 다음과 같은 전처리 과정을 거칩니다.
- 대문자 -> 소문자 변환
- 구두점, 숫자, 특수 문자 제거
- 짧은 단어 제거
# 데이터프레임 생성
news_df = pd.DataFrame({'document': documents})
# 특수 문자 제거
news_df['clean_doc'] = news_df['document'].str.replace("[^a-zA-Z]", " ", regex=True)
# 길이가 3 이하인 단어 제거
news_df['clean_doc'] = news_df['clean_doc'].apply(lambda x: ' '.join([w for w in x.split() if len(w) > 3]))
# 소문자 변환
news_df['clean_doc'] = news_df['clean_doc'].apply(lambda x: x.lower())
# 전처리된 문서 확인
print(news_df['clean_doc'][1])
- 불용어 제거
불용어(stopwords)를 제거하여 의미 없는 단어들을 필터링합니다.
# NLTK에서 불용어 다운로드
nltk.download("stopwords")
stop_words = stopwords.words('english')
# 토큰화 및 불용어 제거
tokenized_doc = news_df['clean_doc'].apply(lambda x: x.split())
tokenized_doc = tokenized_doc.apply(lambda x: [item for item in x if item not in stop_words])
# 전처리된 토큰 확인
print(tokenized_doc[1])
4) TF-IDF 벡터화 및 단어 집합 생성
LDA 모델을 학습하기 위해 TF-IDF 벡터화와 단어 집합을 생성합니다.
# Gensim의 사전 객체 생성
dictionary = corpora.Dictionary(tokenized_doc)
print(dict(dictionary))
# 각 문서를 BOW(Bag of Words) 벡터로 변환
corpus = [dictionary.doc2bow(text) for text in tokenized_doc]
print(corpus[0])
# TF-IDF 모델 생성 및 적용
tfidf_model = gensim.models.TfidfModel(corpus)
corpus_tfidf = tfidf_model[corpus]
# 첫 번째 문서의 TF-IDF 값 출력
for doc in corpus_tfidf:
print(doc)
break # 첫 번째 문서만 출력
# 단어와 TF-IDF 값 출력
for idx, value in doc:
print(f"단어: {dictionary[idx]}, TF-IDF 값: {value}")
5) LDA 모델 학습
TF-IDF 기반의 코퍼스를 사용하여 LDA 모델을 학습합니다.
# LDA 모델 파라미터 설정
NUM_TOPICS = 20
ldamodel_tfidf = gensim.models.ldamodel.LdaModel(
corpus_tfidf, # 입력 데이터 (TF-IDF 기반 코퍼스)
num_topics=NUM_TOPICS, # 토픽 개수
id2word=dictionary, # 단어 사전
passes=15, # 전체 데이터셋 반복 횟수
iterations=400, # 각 문서에서 반복하는 샘플링 횟수
alpha='auto', # Alpha 하이퍼파라미터
eta='auto', # Beta (Eta) 하이퍼파라미터
random_state=42, # 난수 시드 (재현성 확보)
chunksize=2000, # 한 번에 처리할 문서 수
update_every=1, # 모델 업데이트 빈도
minimum_probability=0.01, # 출력할 주제의 최소 확률
per_word_topics=True # 단어별 주제 할당 여부
)
# 학습된 토픽 출력 (각 토픽의 상위 4개 단어)
topics_tfidf = ldamodel_tfidf.print_topics(num_words=4)
for topic in topics_tfidf:
print(topic)
*주요 파라미터 설명
- corpus_tfidf: TF-IDF로 변환된 문서 코퍼스.
- num_topics: 추출할 토픽의 개수.
- id2word: 단어 ID와 실제 단어 사이의 매핑을 정의한 사전.
- passes: 모델이 전체 코퍼스를 몇 번 반복하여 학습할지 설정.
- iterations: 각 문서에서 반복하는 샘플링 횟수.
- alpha & eta: 토픽과 단어의 분포를 조절하는 하이퍼파라미터.
- random_state: 난수 시드 설정으로 재현성 확보.
- chunksize: 한 번에 처리할 문서 수.
- minimum_probability: 출력할 주제의 최소 확률.
# 특정 토픽의 상위 단어 확인
ldamodel_tfidf.print_topics(num_words=2)
topic_number = 0
topic_topn = 4
ldamodel_tfidf.show_topic(topic_number, topn=topic_topn)
6) 시각화
* pyLDAvis
pyLDAvis는 LDA 모델의 결과를 시각적으로 탐색할 수 있게 도와주는 라이브러리입니다.
pip install pyLDAvis
import pyLDAvis.gensim_models
import pyLDAvis
# LDA 시각화
pyLDAvis.enable_notebook()
vis_tfidf = pyLDAvis.gensim_models.prepare(ldamodel_tfidf, corpus_tfidf, dictionary)
pyLDAvis.display(vis_tfidf)
pyLDAvis를 사용하면 각 토픽의 단어 분포, 토픽 간의 거리 등을 시각적으로 확인할 수 있습니다.
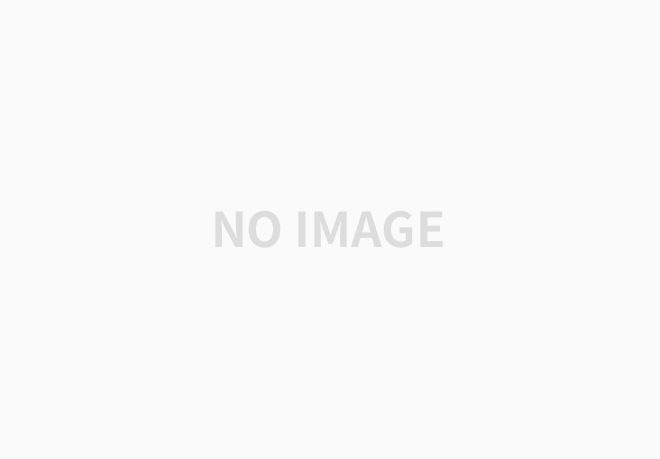
* WordCloud
워드 클라우드는 각 토픽의 단어들을 시각적으로 표현하는데 유용합니다.
pip install wordcloud
from wordcloud import WordCloud
import matplotlib.pyplot as plt
from PIL import Image
import numpy as np
# 예시: 0번 토픽의 상위 5개 단어
topic_0 = [
('data', 0.02), ('science', 0.015), ('machine', 0.012),
('learning', 0.010), ('algorithm', 0.008), ('model', 0.007),
('analysis', 0.006), ('statistics', 0.005), ('intelligence', 0.004),
('python', 0.003)
]
# 워드 클라우드 생성
wordcloud = WordCloud(
width=3000,
height=2000,
random_state=123,
background_color="white",
colormap="Set2",
collocations=False,
stopwords=STOPWORDS
).generate_from_frequencies(dict(topic_0))
# 시각화
plt.figure(figsize=(10, 10))
plt.imshow(wordcloud, interpolation='bilinear')
plt.axis("off")
plt.show()
- 토픽별 워드 클라우드 생성 함수
def plot_wordcloud(lda_model, num_topics, num_words=10):
for t in range(num_topics):
plt.figure(figsize=(8, 8))
plt.imshow(WordCloud(background_color='white').generate_from_frequencies(
dict(lda_model.show_topic(t, num_words))))
plt.axis("off")
plt.title(f"Topic #{t}")
plt.show()
# 토픽별 워드 클라우드 그리기
plot_wordcloud(ldamodel_tfidf, NUM_TOPICS, num_words=10)
- 전체 토픽 기반 워드 클라우드
전체 토픽에서 단어 가중치를 합산하여 전체적인 단어 분포를 시각화합니다.
from collections import defaultdict
# 전체 토픽에서 단어 가중치 합산
word_weights = defaultdict(float)
for topic_id in range(NUM_TOPICS):
topic = ldamodel_tfidf.show_topic(topic_id, topn=100) # 각 토픽에서 상위 100개 단어 추출
for word, weight in topic:
word_weights[word] += weight # 단어의 가중치 누적
# 워드 클라우드 생성
wordcloud = WordCloud(width=800, height=600, background_color='white').generate_from_frequencies(word_weights)
# 시각화
plt.figure(figsize=(10, 8))
plt.imshow(wordcloud, interpolation='bilinear')
plt.axis("off")
plt.show()
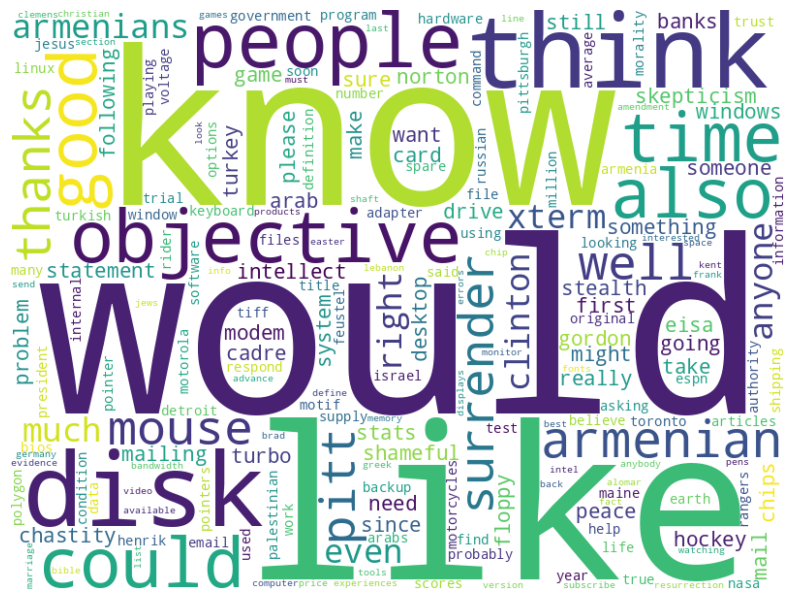
7) 문서별 토픽 분포 확인
각 문서가 어떤 토픽에 얼마나 기여하는지 확인할 수 있습니다.
# 특정 문서의 토픽 분포 확인
print(ldamodel_tfidf[corpus][0])
# 첫 5개 문서의 토픽 분포 출력
for i, topic_list in enumerate(ldamodel_tfidf[corpus]):
if i == 5:
break
print(f"{i}번째 문서의 토픽 비율:", sorted(topic_list, key=lambda x: x[1], reverse=True))
2. ABC News Headlines 데이터를 통한 LDA 분석 (Scikit-learn)
이번에는 ABC News Headlines 데이터를 활용하여 Scikit-learn의 LatentDirichletAllocation을 사용해 LDA 분석을 수행해보겠습니다.
1) 데이터 로드
ABC News Headlines 데이터를 다운로드하고 로드합니다.
import pandas as pd
import urllib.request
# 데이터 다운로드
urllib.request.urlretrieve(
"https://raw.githubusercontent.com/ukairia777/tensorflow-nlp-tutorial/main/19.%20Topic%20Modeling%20(LDA%2C%20BERT-Based)/dataset/abcnews-date-text.csv",
"abcnews-date-text.csv"
)
# 데이터 로드
data = pd.read_csv('abcnews-date-text.csv', on_bad_lines='skip')
print('뉴스 제목 개수 :', len(data))
# 데이터 확인
print(data.head(5))
뉴스 기사 제목만 추출
# 뉴스 기사 제목만 추출
text = data[['headline_text']]
print(text.head(5))
2) 데이터 전처리
뉴스 제목을 전처리하여 모델에 적합한 형태로 변환합니다.
- 토큰화
- 표제어 추출 (Lemmatization)
- 길이가 3 이하인 단어 제거
import nltk
from nltk.stem import WordNetLemmatizer
# 필요한 NLTK 데이터 다운로드
nltk.download('punkt')
nltk.download('wordnet')
# 토큰화
text['headline_text'] = text.apply(lambda row: nltk.word_tokenize(row['headline_text']), axis=1)
# 표제어 추출
lemmatizer = WordNetLemmatizer()
text['headline_text'] = text['headline_text'].apply(lambda x: [lemmatizer.lemmatize(word, pos='v') for word in x])
# 길이가 3 이하인 단어 제거
tokenized_doc = text['headline_text'].apply(lambda x: [word for word in x if len(word) > 3])
print(tokenized_doc[:5])
3) TF-IDF 벡터화
전처리된 텍스트 데이터를 TF-IDF 벡터화합니다.
from sklearn.feature_extraction.text import TfidfVectorizer
# 역토큰화 (토큰화 작업을 되돌림)
detokenized_doc = [' '.join(doc) for doc in tokenized_doc]
# TF-IDF 벡터화
vectorizer = TfidfVectorizer(stop_words='english', max_features=1000)
X = vectorizer.fit_transform(detokenized_doc)
# TF-IDF 행렬의 크기 확인
print('TF-IDF 행렬의 크기 :', X.shape)
# 첫 번째 벡터 출력
print(X[0])
4) 토픽 모델링
Scikit-learn의 LatentDirichletAllocation을 사용하여 LDA 모델을 학습합니다.
from sklearn.decomposition import LatentDirichletAllocation
# LDA 모델 정의
lda_model = LatentDirichletAllocation(
n_components=10, # 추출할 토픽의 수
learning_method='online', # 학습 방법
random_state=777, # 난수 시드
max_iter=1 # 최대 반복 횟수
)
# 모델 학습
lda_top = lda_model.fit_transform(X)
# 학습된 토픽의 크기 확인
print(lda_top.shape)
print(lda_top[0])
# LDA 모델의 단어 집합 확인
terms = vectorizer.get_feature_names_out()
# 토픽별 상위 단어 출력 함수
def get_topics(components, feature_names, n=5):
for idx, topic in enumerate(components):
print(f"Topic {idx+1}:", [(feature_names[i], round(topic[i], 2)) for i in topic.argsort()[:-n - 1:-1]])
# 토픽 출력
get_topics(lda_model.components_, terms)
5) 시각화
* pyLDAvis
pyLDAvis를 사용하여 LDA 모델의 결과를 시각화합니다.
pip install pyLDAvis==3.2.2
import pyLDAvis
import pyLDAvis.sklearn
import numpy as np
# LDA 시각화 설정
pyLDAvis.enable_notebook()
# 단어 목록 추출
feature_names = vectorizer.get_feature_names_out()
# 문서 길이 계산 (TF-IDF 값의 합으로)
doc_lengths = np.asarray(X.sum(axis=1)).flatten()
# 전체 단어 빈도 계산
term_frequency = np.asarray(X.sum(axis=0)).flatten()
# pyLDAvis 데이터 준비
vis_data = pyLDAvis.prepare(
topic_term_dists=lda_model.components_, # LDA 모델의 각 토픽에 대한 단어 분포
doc_topic_dists=lda_top, # 문서별 토픽 분포
doc_lengths=doc_lengths, # 각 문서의 길이
vocab=feature_names, # 단어 목록
term_frequency=term_frequency # 전체 단어 빈도
)
# 시각화
pyLDAvis.display(vis_data)
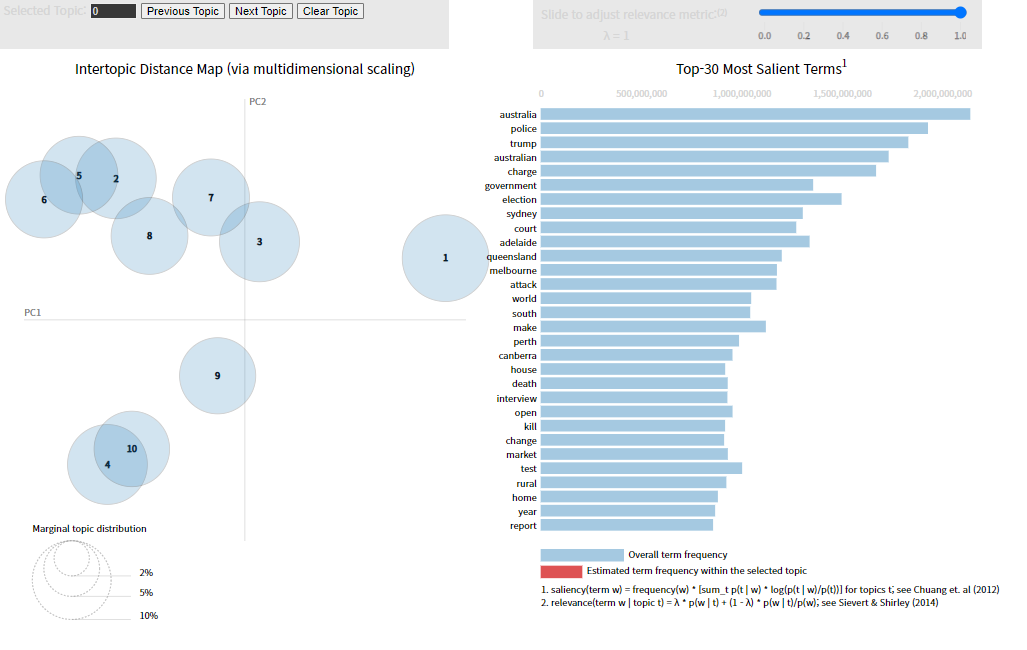
이번 글에서는 Twenty Newsgroups와 ABC News Headlines 데이터를 활용하여 LDA 분석을 수행하는 과정을 살펴보았습니다. 데이터 전처리부터 모델 학습, 시각화까지의 전 과정을 단계별로 따라가며, Gensim과 Scikit-learn을 활용한 두 가지 접근 방식을 경험할 수 있었습니다.
- Gensim을 사용한 분석에서는 TF-IDF 기반의 코퍼스를 통해 LDA 모델을 학습하고, pyLDAvis와 WordCloud를 활용하여 토픽을 시각화했습니다.
- Scikit-learn을 사용한 분석에서는 뉴스 헤드라인 데이터를 전처리한 후, LatentDirichletAllocation을 통해 토픽 모델링을 수행하고 pyLDAvis로 시각화했습니다.
'+ 개발' 카테고리의 다른 글
Word2Vec 모델 만들기 (7) | 2024.09.16 |
---|---|
임베딩 기법 비교: Word2Vec, GloVe, FastText. (3) | 2024.09.15 |
뉴스 데이터 LSA 분석(ft. Numpy & Scikit-learn) (1) | 2024.09.13 |
토픽 모델링(Topic Modeling)의 이해와 활용 (0) | 2024.09.12 |
리스트 컴프리헨션(List Comprehension) (0) | 2024.09.11 |